Agriculture is surrounded by all types of possible issues, including climate change, resource scarcity, and a growing global population that demands increased food production with minimal environmental impact. The evolution of technology, more precisely Artificial intelligence (AI) and robotics, are emerging as transformative solutions, offering data-driven precision, automation, and enhanced decision-making capabilities. These innovations are reshaping conventional farming.
This article examines the integration of AI and robotics in agriculture, analyzing their impact on efficiency, sustainability, and economic viability. By exploring real-world applications, technological advancements, and existing challenges, we provide an overview of these impacts on old and new practices.
The evolution of robotics in agriculture
For a long time, there has been concern about whether food production will be able to supply food to the entire population, which is continuously growing. Initial projections indicated that at some point it would not be possible, but time has fortunately shown otherwise. Both farmers and fishermen have been increasing their production, even at a rate higher than the population growth. Initially, the increase in production was strongly associated with more land use, but in the mid-20th century, this trend began to change. As shown in the figure below, global land use for agriculture peaked in 2000 and decreased since then. On the other hand, the global agricultural production has continued to increase despite this reduction in land use, as can be seen in the following figure:
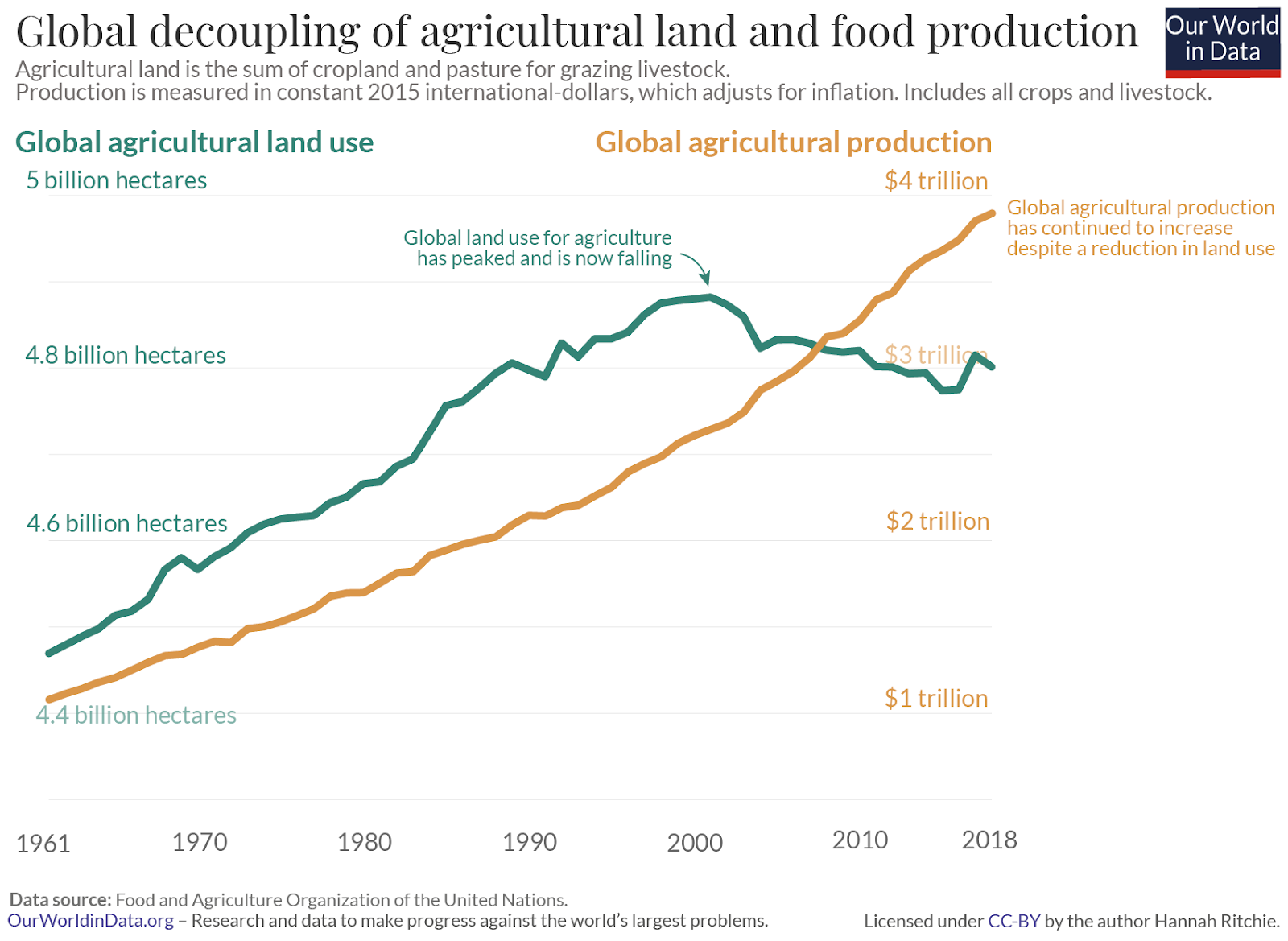
Despite minimal land use expansion, the dramatic increases in food production are a direct result of technological advancements. This transformative process began in the early 20th century with the introduction of tractors and other machinery, marking the onset of what is commonly referred to as the “Green Revolution”. Known also as the Third Agricultural Revolution, the Green Revolution was a period of major technological advancements that led to vastly improved crop yields. Initially implemented by developed countries in the early 20th century, it was later adopted worldwide, particularly for extensive crops.
Following the Green Revolution, we are now witnessing the rise of the Fourth Agricultural Revolution, driven by advanced technologies like AI, robotics, and data analytics. The Fourth Agricultural Revolution marks a new chapter in farming, one that is heavily reliant on cutting-edge technological innovation. But before discussing how robotics enables this revolution, let's briefly discuss the different types of agricultural production.
Types of Agricultural Productions
The specific type of agricultural production heavily influences the design and application of agricultural robots, much like how agronomical engineers and skilled workers specialize in particular areas of agriculture.
Different agricultural production types have different cost distributions throughout their lifecycles. In the case of an olive orchard, for instance, the initial planting is a significant investment that lasts for many years (30-40 years), whereas extensive annual crops require planting every year. This disparity in costs across different production stages directly impacts technology implementation.
Extensive Crop
Extensive field crops, such as wheat, corn, and soybeans, are grown over large areas. The main characteristic of this type of production is that it's an annual crop, which means that the entire lifecycle is repeated annually: seeding, growing, and harvesting.
.jpg)
Land Preparation and Seed Sowing
Land Preparation and Seed Sowing are the first steps in the process. In some cases, Land Preparation implies tiling, but in other cases, such as direct sowing, the land preparation is not even necessary. These processes are strongly technified: nearly all the land preparation and seed sowing for extensive crops is done using specialized machinery (although not autonomous: all of them are driven by humans).
Monitoring
Once the crop has been seed, it usually requires monitoring the different variables that impact its normal development. Activities such as controlling weeds, pests and diseases, water and fertilization requirements are key to obtaining good crops. In all these activities, the use of technology is crucial as we will see in the next sections.
Harvesting
Nearly in any extensive crop production, the harvesting is fully automated using specialized machinery called combine harvester. The combine harvester, a crucial piece of agricultural machinery, is designed for efficiently harvesting various cultivated seeds. By integrating multiple harvesting steps—reaping, threshing, and winnowing—into one operation, it became a major labor-saving innovation in the early 20th century, dramatically decreasing the agricultural workforce.
Orchards
Although the biological lifecycle of an orchard mirrors that of other plants, a primary distinction lies in its extended lifespan. Orchards, once established, persist for multiple years. Fruit-bearing may eventually cease, and the harvesting process is typically more intricate and requires greater care to prevent fruit damage, particularly with sensitive varieties such as cherries. These distinctions contribute to a unique cost profile compared to extensive crop production.
Land Preparation and Planting
Orchards are typically established using saplings that have been pre-grown in a greenhouse or nursery. This practice ensures a higher survival rate and allows for better initial growth and health compared to planting seeds directly. Each tree is planted with meticulous care, as it represents a long-term investment, expected to yield fruit for several years, often decades. The planting process involves preparing the soil with the necessary nutrients, spacing the trees appropriately for optimal growth and sunlight exposure, and providing adequate support and initial watering. Due to the long lifespan and substantial investment associated with orchards, careful planning and execution of the planting phase are crucial for the orchard's future productivity and economic viability.
Monitoring
Monitoring an orchard presents a unique set of advantages and challenges. On the one hand, the significant investment required to establish and maintain an orchard makes it economically justifiable to invest in advanced technologies for monitoring its health. This can lead to early detection of issues, optimized resource allocation, and improved overall orchard productivity. On the other hand, the specific technologies employed for orchard monitoring can vary widely in their complexity and cost. Simple visual inspections might be sufficient for some situations, while others may require sophisticated sensor networks, drone imagery, or AI-powered analytics. The choice of technology depends on the scale of the orchard, the type of fruit, the level of desired precision, and the available budget. Moreover, the interpretation of data from these technologies requires specialized knowledge, adding another layer of complexity.
Forestry
Similarly to the previous production, Forestry has distinct stages: planting, growth, and harvesting. Each stage requires different management and resources. As in the case of orchards, forestry involves a long-term commitment. Trees are planted with the expectation of growth over many years before they can be harvested.
Planting
Planting is typically done using saplings pre-grown in a greenhouse or nursery. This method improves survival rates and initial health. Trees are often planted in lines with specific spacing to optimize growth and management.
Monitoring
Young trees, like Eucalyptus saplings, face competition for resources such as water and nutrients, especially from weeds. This competition can significantly impact their survival and growth. Thus, weed control is a crucial aspect of forestry. It's often done through herbicide application, but there's a growing interest in more targeted and sustainable methods.
Harvesting
Forestry harvesting is a long-term operation that requires careful planning and execution. Unlike harvesting in annual crops, trees take many years to mature, making the timing and method of harvest crucial. The process begins with planning, considering factors like tree age, species, and market demand, ensuring sustainable cycles and forest regeneration. Various methods exist, including clear-cutting, selective cutting, and thinning, each with distinct environmental impacts and management goals. Specialized equipment such as feller bunchers, skidders, processors, and harvesters are used to cut, delimb, and transport logs. Logistics and transportation play a vital role, often involving road building and the use of trucks or trains. Throughout the process, environmental considerations are paramount, with a focus on sustainability, reforestation, biodiversity, and protecting soil and water quality. Certifications like Forest Stewardship Council (FSC) and the Programme for the Endorsement of Forest Certification (PEFC) indicate responsible forestry practices. Technologies like GPS, GIS, and remote sensing aid in planning and monitoring, while there's growing interest in automated machinery despite the complex terrain and weather challenges.
Greenhouses
Greenhouses offer a unique environment for vegetable production, distinct from both extensive field crops and orchards. While the fundamental plant lifecycle remains the same, greenhouse production allows for a much more controlled and often automated approach. This environment enables the cultivation of a wide variety of vegetables, but it particularly favors crops that require specific temperature, humidity, and light conditions. Common greenhouse vegetables include tomatoes, cucumbers, lettuce, peppers, and herbs. The enclosed space of a greenhouse, even large-scale ones, is significantly smaller than an open field, making it ideal for fully automating certain processes such as irrigation, nutrient delivery, and even harvesting in some cases. This controlled setting allows for optimized growing conditions, leading to higher yields and better quality produce. The type of vegetable production implemented in a greenhouse is generally intensive, focusing on maximizing output per unit area.
Types of agricultural robots
Demographic growth and climate change are placing increasing pressure on regional agricultural production. The challenge of increasing crop yields while ensuring the sustainable development of environmentally friendly agriculture is a common problem worldwide. Automated systems, remote sensing services & technology, and major advances in artificial intelligence offer great opportunities to address this issue right now.
In recent years, artificial intelligence in all its variations has shown great potential to reshape many industries, mainly due to its powerful ability to learn from massive datasets, and the agricultural industry is no exception. Currently, there is an attempt to convert traditional precision agriculture into data-driven precision agriculture, with the aim of applying the correct treatment, in the right place, at the right time, taking into account the spatiotemporal variabilities of farmland.
Within the context of precision agriculture, the scientific community has shown great interest in recent years in transferring advances in deep learning from non-agricultural to agricultural applications. Specifically, using deep learning at different scales during the crop growth cycle, for the identification and quantification of diseases and pests at the leaf scale; the classification and identification of plants and weeds at the canopy scale; the assessment of abiotic stress, plant growth, nutrient status monitoring, and yield prediction at the field scale; while at the regional scale it can be implemented for coverage mapping, regionalization of soil status, crop discrimination, among others.
Although there are several satellites that provide high spatial resolution images (<2m) and temporal resolution (daily), most publicly available (free access) satellite products do not provide sufficiently fine information for many precision agriculture applications. The appropriate spatiotemporal resolution for precision agriculture depends on several factors, including management objectives, field size, and the ability of agricultural equipment to vary the application rates of inputs (irrigation, fertilizers, pesticides, etc.). For example, the estimation of biomass and crop yield generally requires a spatial resolution of approximately 1 to 3 meters, and for irrigation and fertilizer applications, it is estimated between 5 and 10 meters. Furthermore, weed mapping and streamlined herbicide application require an even finer spatial resolution, between 5 and 50 centimeters, depending on the size of the weed patches (Sichodia, 2020).
Aerial platforms (like drones) generally provide images with higher spatial resolution (on the centimeter scale) compared to satellites, but they are limited in their autonomy and therefore their area of use. The correct choice between using satellite images and images taken by UAVs is fundamental to the success of the project. Today, a lot of work is being done on integrating both types of images to address the weaknesses of each one separately.
As a complementary option, vision systems can be placed on agricultural vehicles, such as tractors or sprayers. These can collect data on field operations, which can be used to train AI methods. This approach allows for redefining the system by adding AI without significantly changing the operating mode that is already in place.
The following sections provide examples of robots used in agriculture.
Planting Robots
Planting robots vary widely depending on the type of agricultural production. They include autonomous tractor robots, which are driverless tractors with advanced navigation systems for precise planting; drone planters, used for dispersing seeds over large or hard-to-reach areas, ideal for reforestation; and robotic arm planters, designed for delicate tasks like transplanting seedlings, particularly useful in nurseries and greenhouses. The market for planting robots is experiencing substantial growth, indicating increasing adoption of automation in agriculture, with the global market estimated at $13.5 billion USD in 2023 and a projected annual growth rate of 24.3% through 2028.
Monitoring Robots
Robots used for inspection and monitoring in agriculture offer farmers valuable information about various factors such as soil moisture, pollution levels, fertilization effectiveness, pest presence, and plant diseases. These robots collect readings, examine plants for signs of blight, determine optimal harvest times, and ultimately contribute to improved crop yields. They are employed in diverse settings, including open fields and controlled greenhouse environments, where they monitor parameters like greenhouse gas concentrations and humidity levels.
Depending on the type of production, these robots may have different characteristics. For instance, unmanned vehicles are more easily deployed on greenhouses and nurseries. In these productions, the environment is usually well controlled, which simplifies the requirements for a robot.
Although many inspection tools are ground-based for close-up analysis, aerial drones also play a significant role in data collection. These drones gather information about crop health and growth stages, which empowers farmers to optimize yields, lower costs, and enhance sustainability. Given the increasing global food demand, agricultural robots are vital for boosting efficiency, yield, and environmental sustainability, enabling the industry to meet rising needs while tackling emerging challenges.
The role of AI in agricultural robotics
Artificial Intelligence (AI) is often a vague and poorly defined term, frequently associated with various techniques used by researchers in the field, such as deep learning, reinforcement learning, and machine learning. Due to its emergence as a highly profitable industry, many things get classified as AI. A recent study by Vincent et al. reveals that over 40% of 'new AI companies' don't even use AI. Therefore, clearly defining and distinguishing what constitutes AI is crucial to analyzing its impact on the agricultural sector.
Initially, AI applications within the agricultural sector can be divided into AI-powered software and AI-powered robots. AI software is essential for the operation of AI robots, but AI robots are not (generally) essential for the operation of AI software.
Robots have been used in agriculture for quite some time. For example, robotic milking systems have been around for over two decades. However, the use of agricultural robots powered by artificial intelligence (AI) is a relatively recent development. These AI-driven agricultural robots can perform a wide variety of tasks, including crop scouting, pest and weed control, harvesting, spraying, pruning, milking, phenotyping, and sorting. These robots must be able to adapt to their surroundings and navigate complex terrains, often amongst the crops themselves. Currently, most AI robots are still in early stages of development, with many being used in test facilities, projects, and research centers. Very few have reached a commercial stage, and most are not yet able to match the speed of standard equipment or human workers.
AI is also used directly through software applications. Image recognition enables farmers to determine the health or disease of a specific plant or crop and provides recommendations on what actions to take. Some AI systems map the life stages and growth of plants, predicting how they will change throughout their cycle and suggesting necessary actions for the farmer. In this way, AI is replacing many tasks traditionally performed by agronomists and allowing farmers to streamline management and decision-making in the field. Companies such as BASF, Monsanto, Bayer, Pioneer, and John Deere are already utilizing data collected from fields to provide personalized information and recommendations to farmers, with the aid of AI technologies. This is not entirely new and is often referred to as precision agriculture. However, what is new in agricultural AI are the tools and types of innovation used to achieve these goals. In general, AI provides information on what a farmer should do, when, how, and to what extent.
Case studies: successful implementations
Precision Agriculture
Perhaps one of the most successful implementations of technology in a production environment is Precision Agriculture. As defined by the “International Society of Precision Agriculture”, Precision Agriculture is a management strategy that gathers, processes and analyzes temporal, spatial and individual plant and animal data and combines it with other information to support management decisions according to estimated variability for improved resource use efficiency, productivity, quality, profitability and sustainability of agricultural production.
It is strongly supported by the geolocalization capabilities of the machinery used in extensive crops. With the incorporation of GPS, the farmer's and/or researcher's ability to locate their precise position in a field allows for the creation of maps of the spatial variability of as many variables as can be measured. Another improvement in this machinery was the incorporation of Variable Rate Technology (VRT). VRT is a key part of precision agriculture. It allows farmers to adjust the amount of resources they apply to different parts of their field, which maximizes crop yield and minimizes waste. This targeted approach also reduces the negative impact on the environment compared to applying the same amount of resources across the entire field. These two technologies are combined in a powerful way. First of all, a detailed map of the field for the specific variable of interest is done. This is usually done by means of satellite or drone images, where a certain variable of interest is analyzed. For example, using KMeans to create clusters where the NDVI is homogeneous. With this map, a farmer can decide for example, the amount of fertilizer to apply to each different cluster. Because of the capabilities of the machinery that have GPS and VRT, the process of applying the correct amount of fertilizer is automatic with no intervention of the farmer. The only human process is to drive the machinery.
The capabilities of VRT and GPS are used also at harvesting and again has an enormous impact in agriculture production. Yield monitors measure crop yield and the location in the field in real time. This is useful not only to plan for the logistics of the crop production but also to analyze the data and to find patterns.
Weed Detection and Control
Weed control is a very important management task, aimed at preventing the reduction of crop yield, and is usually addressed through mechanical treatments or the application of herbicides. However, mechanical treatment is often complicated to carry out and ineffective if not done properly, which makes the application of herbicides the most widely used option. However, using large amounts of herbicides entails significant costs and produces harmful effects on the environment, especially when applied uniformly, without considering the spatial distribution of the weeds.
Control based on artificial vision and deep learning offers a promising opportunity to manage weeds effectively (especially to mitigate the generation of herbicide resistance due to misuse), through the identification and location of weeds, followed by specific in situ treatments (e.g., spot spraying or by sectors and mechanical removal of weeds). While solutions already exist in production, developing systems capable of recognizing weeds with high precision under changing light and soil conditions remains a critical challenge.
Unmanned aerial vehicles (UAVs) have been the most popular remote sensing platform for weed mapping and management, mainly due to their ability to produce images with centimeter-scale resolution. However, the commercial adoption of these technologies remains a challenge, given the necessary expertise for operation and the processing capacity required for the data obtained.
As an alternative, solutions can be found that involve placing cameras and computers on operational machinery in order to perform detection using computer vision. This form of implementation presents challenges at the level of the materials used. With the advent of artificial intelligence, more and more cameras are suitable for working in field conditions and can provide high-resolution images at a sufficiently high rate. Similarly, the range of computers that support use in field conditions and have sufficient processing capacity to run these artificial vision algorithms, such as those based on real-time deep learning, has been expanding.
In this context, weed detection can be approached by installing a vision system as close to ground level as possible to obtain better images of the weed situation. Thus, object detection and segmentation methods allow for the spatial detection of weeds and their subsequent treatment with herbicide. For example, the following image shows the system installed by Digital Sense for detecting weeds in Eucalyptus plantations.
The system consists of a camera, lighting, and a tent that protects the camera from collisions with the Eucalyptus crops. For this vision system, it is important to control the scene's lighting, so the tent was covered with diffuser fabric, and attempts were made to lower the tent as much as possible without compromising the health of the crops.


Irrigation for the forestry industry
When a forest is planted, and during the first years, irrigation is key to the survival and correct installation of the forest. Because the irrigation is done only in the first years of the forest installation, and also because of the size of the plantation, a fixed irrigation system is prohibitively expensive. In most cases, irrigation is performed with a tank attached to a tractor. The driver applies the water, which is obviously not optimal: the driver has to drive in addition to looking for the tree to apply the water and can only process one line at a time. At Digital Sense, we have developed an automatic irrigation system. This system, composed of several cameras looking at several tree lines simultaneously, is linked to the irrigation system, and each time a tree is detected, a signal is sent to the irrigation system to water the corresponding tree.
Benefits of using robots in agriculture
The benefits of using automation on agricultural production activities will depend on the type of processes and tools we are considering. The main benefits can be grouped as follows:
During Monitoring Process
Usually, monitoring is done by human inspection, with the farmers and technicians visiting the field and analyzing what they see. They can also support the analysis with some tools (to measure soil moisture or temperature). A second step in incorporating technology is to provide the technician with tools to better analyze the field, both in terms of quality and time. For instance, the use of mobile apps to analyze plant stress or to
One key benefit of adding technology to the monitoring process is to perform data capture unattended. This can be done in several ways, but one basic piece of valuable information is to incorporate sensors to monitor temperature, wind direction and velocity, rain, and other valuable climatological data. Usually, these sensors can take these measures hourly, generating a lot of information that is used by engineers to better plan the activities in the field.
The use of IoT to obtain real-time information from the field is an obvious benefit.
The use of inspection robots to check the sanity of a crop is also very valuable. Computer vision, using different wavelengths, can detect things that are not even visible to the expert eye. For instance, a crop stress due to a pest may not be visible in the early phases, but the response of the plant to certain segments of the electromagnetic spectrum can be used to detect the stress.
Challenges and limitations
There are several challenges that agricultural robots face. The most relevant are:
- Variability of the environment: Agricultural robots face substantial hurdles due to the dynamic nature of their operating environment. The complexity of navigating through fields and orchards, which often feature irregular terrain, obstacles, and inconsistent soil surfaces, makes reliable operation a considerable challenge. The varying ground conditions can affect robot movement, sensor readings, and overall performance.
- Variability of the climatological conditions: This poses a major challenge for agricultural robots. Systems designed for one region, may not function effectively in another region due to differences in climate, weather patterns, and local environmental factors. These variations can affect robot performance, sensor readings, and overall reliability.
- The cost-effectiveness of agricultural robots must be clearly analyzed. While labor shortages in industrialized nations can drive adoption, in regions with ample labor, simply replacing humans with robots may not be economically viable. Furthermore, in production systems like extensive crops, human labor is often already minimal, primarily involving tractor or machinery operators during specific phases like seeding, herbicide application, or harvesting. Therefore, a thorough understanding of the true cost/benefit ratio is essential to determine whether implementing agricultural robots is beneficial and sustainable.
What does the agriculture of the future look like?
The agriculture of the future, driven by the Fourth Agricultural Revolution, will be fundamentally transformed by technology. Building upon the advancements of the Green Revolution, we will see an increased integration of digital tools, data analytics, AI, and robotics into every aspect of farming. This includes precision agriculture techniques like Variable Rate Technology (VRT) and GPS-guided machinery becoming standard practice. We will see autonomous robots performing tasks such as planting, monitoring, and even harvesting, increasing efficiency and reducing labor needs. AI-powered systems will aid decision-making, optimize resource allocation, and minimize environmental impact. Data collected from sensors, drones, and satellites will be analyzed in real-time, providing valuable insights for farmers.
While challenges like environmental variability and cost-benefit ratios exist, these are being actively addressed. The agriculture of the future will be characterized by increased automation, data-driven decision-making, and a focus on sustainability, ensuring food production can meet the demands of a growing population while minimizing its impact on the planet - for those interested in what is ClimateTech and AI, make sure to check our article.